Businesses are moving beyond generic marketing to predictive segmentation, using data to tailor experiences, boost engagement, and build lasting customer relationships. The predictive analytics market is projecting a surge from $17.07 billion in 2024 and $20.77 billion in 2025 to a staggering $52.91 billion by 2029. As it’s growing at a compound annual growth rate (CAGR) of 26.3%, it is evident that this technology is reshaping the future of marketing.
This comprehensive guide explains predictive segmentation, its benefits, challenges, real-world applications, and strategies for effective implementation to help businesses harness their full potential.
What is Predictive Segmentation?
Predictive segmentation is the practice of using data analytics and machine learning to group customers based on predicted behaviors and preferences.
Unlike traditional segmentation methods, predictive segmentation dynamically processes vast amounts of customer information to create highly accurate and actionable customer profiles.
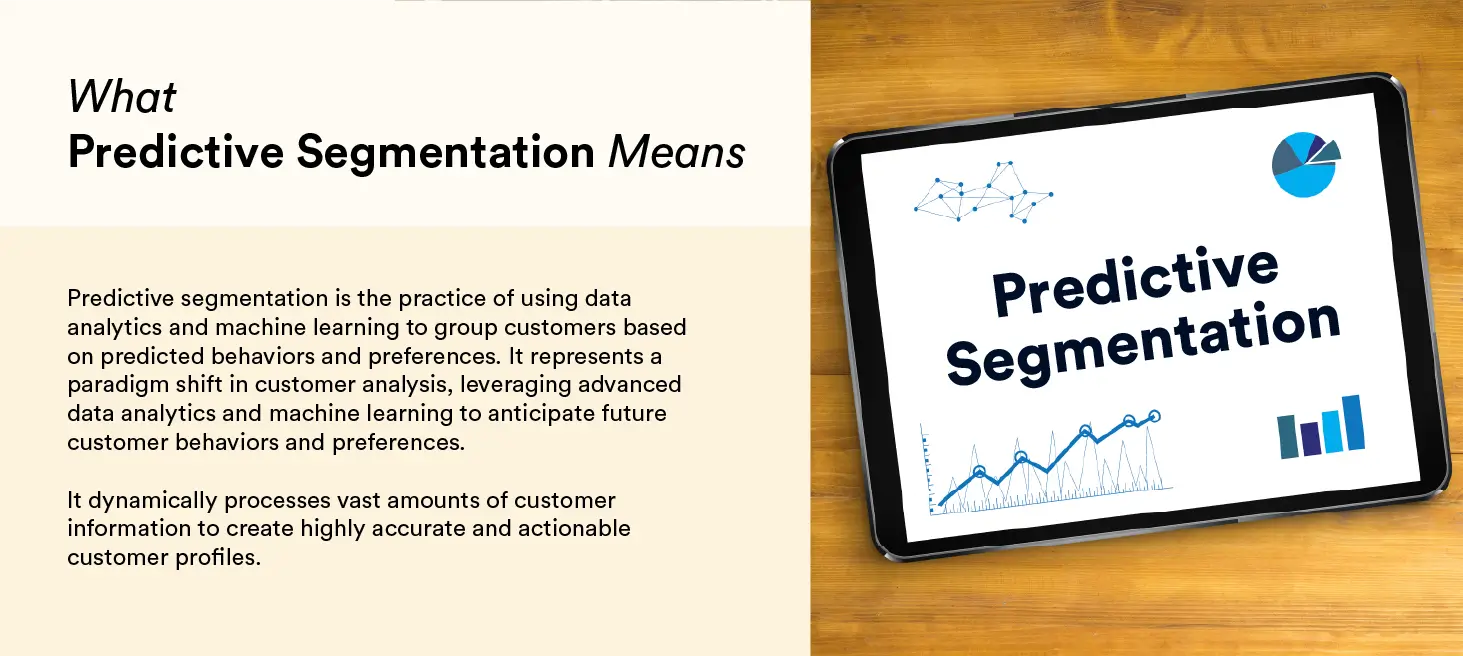
Key Components of Predictive Segmentation:
- Data Analytics: At its core, predictive segmentation utilizes sophisticated statistical algorithms to analyze historical and real-time customer data.
- Machine Learning: Advanced AI models continuously learn from new data, improving the accuracy of predictions over time.
- Diverse Data Sources: Predictive models incorporate a wide range of data types, including:
- Demographic information (age, gender, income)
- Behavioral data (purchase history, website interactions)
- Transactional data (frequency of purchases, average order value)
- Psychographic information (interests, values, lifestyle choices)
- Real-time Processing: Unlike static segmentation methods, this technology can update customer profiles in real time based on the latest interactions and behaviors.
Predictive segmentation synthesizes these elements and creates a multidimensional view of each customer. As a result, businesses can anticipate needs, personalize experiences, and optimize marketing strategies with unprecedented precision.
Benefits of Implementing Predictive Segmentation
Predictive segmentation empowers businesses with deeper insights and enhanced marketing strategies to improve customer engagement. Below are the detailed benefits:
Enhanced Personalization
- Precision-targeted messaging: Businesses can craft highly personalized marketing messages that align with individual customer preferences, behaviors, and purchase history.
- Dynamic content recommendations: AI-driven insights enable platforms to suggest products, services, and content tailored to individual users, increasing conversion rates.
- Real-time customization: Customer interactions update profiles in real time, allowing businesses to respond instantly with relevant offers and promotions.
Increased Marketing ROI
- Resource optimization: Predictive segmentation helps identify high-value customer segments. It ensures that marketing budgets are focused on the most promising leads.
- Reduced marketing waste: Businesses can eliminate irrelevant targeting, improve campaign efficiency, and lower acquisition costs.
- Optimized ad spend: Predictive models enhance ad targeting, ensuring that promotional content reaches the audience most likely to convert. This leads to higher engagement and sales.
Improved Customer Retention
- Early churn detection: AI-driven insights identify customers at risk of leaving by analyzing declining engagement or purchasing patterns.
- Proactive re-engagement: Businesses can deploy personalized retention strategies, such as exclusive offers or loyalty incentives, to win back customers before they churn.
- Tailored customer service: Support teams can leverage predictive segmentation to anticipate customer needs and deliver proactive solutions.
More Accurate Forecasting
- Data-driven decision-making: This segmentation method provides businesses with precise forecasting of future consumer trends, which ensures more informed strategic planning.
- Inventory and demand planning: Retailers and e-commerce platforms can optimize stock levels by predicting demand patterns based on past behaviors and seasonal trends.
- Strategic resource allocation: Businesses can distribute resources more effectively by forecasting market demand and adjusting operational capacities accordingly.
Competitive Advantage
- Deep market insights: Predictive segmentation unveils nuanced customer preferences, allowing businesses to stay ahead of competitors.
- Agility in response to market trends: Companies can quickly adapt their marketing strategies to align with emerging trends and shifting consumer behaviors.
- Product and service innovation: Predictive insights help businesses develop new offerings tailored to evolving customer needs, ensuring sustained relevance in the marketplace.
Challenges and Considerations
While the benefits of predictive segmentation are substantial, businesses must navigate several challenges to implement it successfully:
- Data Quality and Integration
Ensuring data accuracy and consistency across multiple sources is a significant challenge. Businesses must integrate disparate data systems to create a unified customer view, which can be complex and time-consuming. Additionally, maintaining data hygiene is crucial, as poor-quality data can lead to inaccurate insights and ineffective segmentation strategies.
- Privacy and Compliance
Businesses must comply with data protection regulations like GDPR and CCPA, which require strict guidelines on data collection, storage, and usage. Balancing personalization with customer privacy concerns is critical, as overly intrusive data practices can lead to consumer distrust. Implementing robust data governance frameworks ensures compliance while maintaining ethical data practices.
- Technical Expertise
Predictive segmentation relies on advanced analytics and machine learning, requiring skilled professionals to develop and manage these models. Businesses often struggle with acquiring or upskilling talent to keep pace with rapidly evolving technologies. Moreover, translating complex analytical insights into actionable business strategies remains a challenge for many organizations.
- Organizational Alignment
Breaking down silos between marketing, IT, and other departments is essential for successful implementation. A lack of collaboration can lead to fragmented data and inefficient decision-making. Fostering a data-driven culture across the organization and ensuring alignment with overall business objectives can enhance the effectiveness of predictive segmentation.
- Scalability and Real-time Processing
Handling large volumes of data in real time presents a technical challenge, especially as businesses grow. Ensuring system performance and reliability while managing increasing data loads is crucial. Additionally, balancing the need for real-time insights with computational constraints requires robust infrastructure and strategic resource allocation.
To address these challenges, businesses should adopt a strategic approach, investing in robust data infrastructure, fostering cross-functional collaboration, and prioritizing ongoing education and skill development.
Implementing Predictive Segmentation in Your Business
Successfully implementing predictive segmentation requires a structured approach that ensures data readiness, technology alignment, and actionable insights. Follow these key steps:
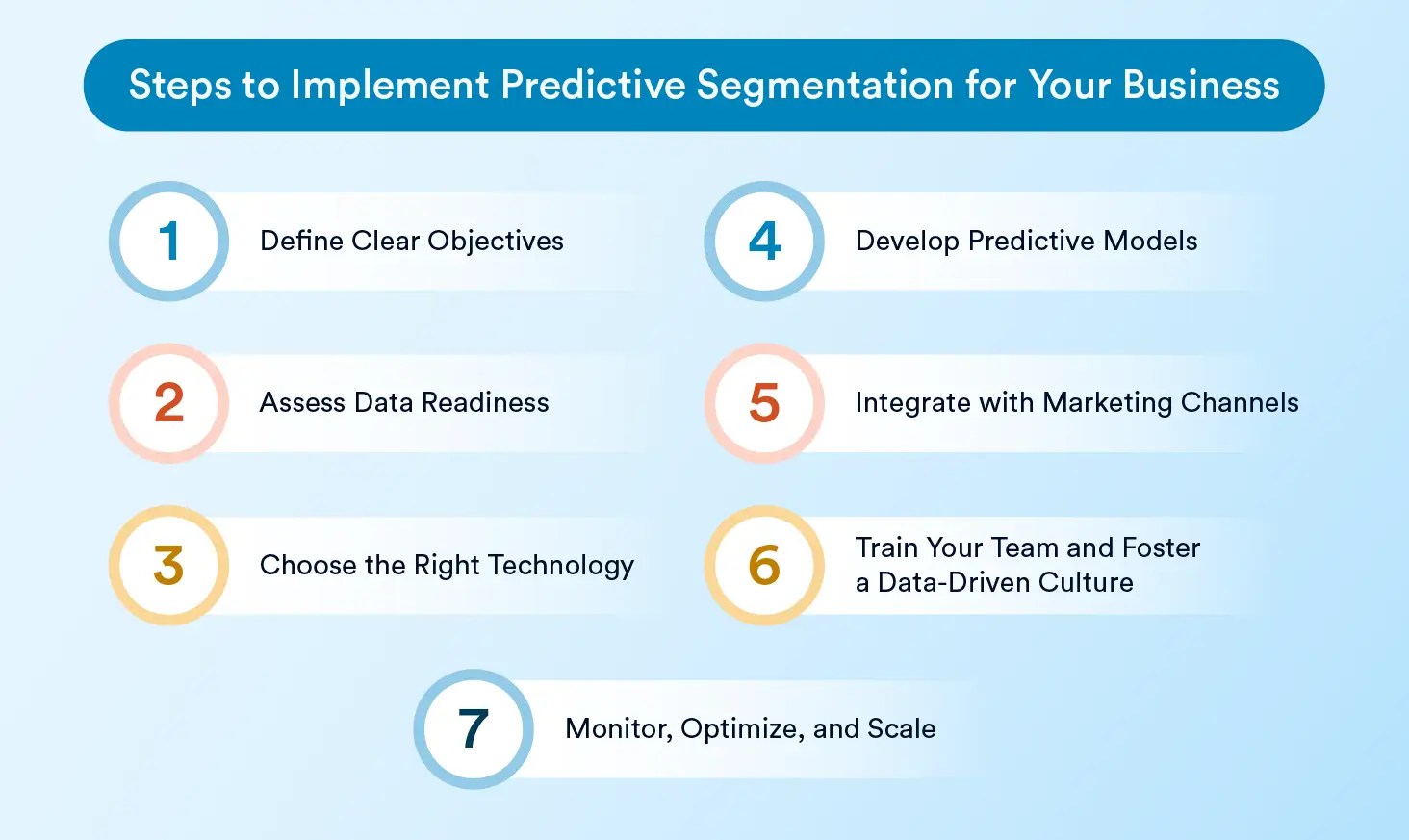
1. Define Clear Objectives
Before diving into predictive segmentation, businesses must outline specific goals and desired outcomes:
- Identify primary objectives, such as increasing customer retention, enhancing personalization, or optimizing marketing spend.
- Align segmentation strategies with overall business and marketing goals.
- Establish measurable key performance indicators (KPIs) to track the success of predictive segmentation initiatives.
2. Assess Data Readiness
Data is the foundation of predictive segmentation, and ensuring its quality and accessibility is critical:
- Conduct a comprehensive audit of available data sources, including CRM, website analytics, transaction logs, and customer feedback.
- Identify gaps in data collection and ensure consistency across different channels.
- Implement data cleansing and enrichment processes to improve data accuracy and reliability.
- Integrate structured (demographics, purchase history) and unstructured data (social media sentiment, customer reviews) for a holistic view.
3. Choose the Right Technology
Selecting the right predictive analytics tools is crucial for successful implementation:
- Evaluate available AI-driven segmentation platforms based on scalability, integration capabilities, and ease of use.
- Consider cloud-based analytics solutions that offer real-time processing and machine learning capabilities.
- Ensure seamless integration with existing marketing automation and customer relationship management (CRM) systems.
- Prioritize platforms that support advanced visualization and reporting for easy interpretation of insights.
Unlock AI-powered segmentation that drives real results with Clever.AI
4. Develop Predictive Models
Creating effective predictive segmentation models involves:
- Selecting appropriate machine learning techniques, such as decision trees, neural networks, or clustering algorithms.
- Starting with simple models and gradually refining them with more complex methodologies.
- Training models using historical data and continuously testing their accuracy with real-time inputs.
- Employing A/B testing to compare different segmentation strategies and measure performance.
5. Integrate with Marketing Channels
Once predictive segments are created, businesses must seamlessly integrate them into their marketing strategies:
- Deploy personalized content and product recommendations across multiple channels (email, SMS, push notifications, in-app messages).
- Ensure real-time decision-making to deliver timely, context-aware marketing messages.
- Implement omnichannel marketing strategies that unify customer interactions across web, mobile, and in-store experiences.
- Leverage predictive insights for dynamic pricing, upselling, and cross-selling opportunities.
6. Train Your Team and Foster a Data-Driven Culture
The success of predictive segmentation depends on the ability of teams to interpret and act on data-driven insights:
- Provide comprehensive training on predictive analytics tools and methodologies to marketing, sales, and customer service teams.
- Encourage collaboration between data science teams and business units to translate insights into actionable strategies.
- Promote a culture of experimentation and continuous learning, empowering teams to optimize segmentation models.
7. Monitor, Optimize, and Scale
Predictive segmentation is not a one-time implementation but an ongoing process that requires regular refinement:
- Continuously monitor the performance of predictive models and adjust strategies based on real-time results.
- Use A/B testing and customer feedback to optimize segmentation accuracy.
- Stay agile and adapt to evolving consumer behaviors, market conditions, and technological advancements.
- Scale predictive segmentation efforts across multiple business units or geographical markets to maximize impact.
By following these steps, businesses can build a robust predictive segmentation framework to enhance customer engagement, improve marketing efficiency, and drive long-term growth.
Powerful Real-World Examples of Predictive Segmentation
Paysend (Fintech, UK)
Paysend, a London-based fintech app for global money transfers, needed to boost user transactions and retention. By leveraging CleverTap’s predictive segmentation, Paysend automatically identified valuable user cohorts and those at risk of churn. Custom events such as registration completion and past behavioral data were used to create segments, such as:
- New users who haven’t transacted within 3 days of signing up
- Loyal users who suddenly became inactive
Paysend then targeted each group with tailored messages and offers to increase engagement and lifetime value.
Results:
- 17% average click-through rate on push notifications (10× industry average)
- 22% increase in weekly app registrations
- 23% rise in repeat money transfers quarter-over-quarter
- 5.4% improvement in first-time user conversion rates
These efforts contributed to a 23% boost in repeat transactions and a significant increase in customer lifetime value (LTV).
How Paysend boosted repeat transactions by 23% using predictive segmentation:
Read the full case studyBlinkit
Blinkit, an India-based online grocery platform, used CleverTap’s predictive segmentation to categorize users by:
- Purchase frequency, recency, and value
- Brand affinity and regional preferences
They implemented real-time segmentation, such as users inactive for 15–30 days, and triggered personalized win-back campaigns via push notifications, SMS, and email.
Results:
- +6% retention rate after personalized re-engagement
- +53% Week-1 new user login rate via automated onboarding
- +2.6% conversion rate from real-time cart-abandonment campaigns
By leveraging predictive insights, Blinkit optimized promotions, boosted repeat purchases, and increased revenue from previously lost opportunities.
How Blinkit re-engaged dormant users and increased week-1 logins by 53%:
Read the full case studyCleverTap’s Predictive Segmentation and Predictive Analytics
CleverTap offers a comprehensive predictive segmentation solution that leverages advanced AI and machine learning capabilities. Key features include:
- Advanced Data Processing:
- Analyzes vast amounts of structured and unstructured data
- Tracks user actions over a 10-year lookback window via TesseractDB™
- Identifies trends and patterns in customer behavior
- Real-Time Segmentation:
- Updates customer segments dynamically based on the latest interactions
- Enables immediate response to changing customer behaviors
- Predictive Analysis and Behavioral Modeling:
- Forecasts future customer actions (e.g., purchases, churn) using historical and live data
- Creates micro-segments using Intent-based segmentation to predict goal completion likelihood
- Identifies high-value, at-risk, or loyal users with RFM segmentation and power user cohorts
- Personalization at Scale:
- Enables hyper-personalized messaging using psychographic, demographic, and behavioral data
- Supports contextual content delivery based on interests, past behavior, and emotional tone via CleverTap’s Scribe
- Automated Decision-Making:
- Optimizes campaign performance using AI-driven journey orchestration with IntelliNode
- Frees marketers to focus on strategy rather than manual data analysis
- Continuously tests variations and adjusts messaging paths for best-performing outcomes
- Enhanced Customer Profiles:
- Integrates data from multiple sources for a comprehensive customer view
- Facilitates more relevant and effective engagement strategies
Drive hyper-personalized growth through predictive insights with CleverTap
To implement CleverTap’s predictive segmentation:
- Define clear segmentation goals aligned with business objectives
- Capture and unify high-quality user data from all customer touchpoints
- Leverage prebuilt predictive models (e.g., churn likelihood, conversion intent)
- Monitor campaign impact using Real Impact Dashboard
- Continuously refine segments and engagement strategies with insights from cohorts, pivots, and A/B tests
Businesses leveraging these advanced capabilities can create highly targeted marketing campaigns that resonate with individual customers, driving engagement and loyalty.
Future Trends in Predictive Segmentation
As we approach 2025 and beyond, several key trends are reshaping predictive segmentation. Businesses that adapt quickly will deliver smarter, faster, and more relevant customer experiences.
- AI-Powered Hyper-Personalization
Advanced AI models now enable highly granular customer segmentation. Real-time personalization is becoming standard across digital and physical touchpoints, allowing brands to respond instantly to customer behavior.
- Integration of Zero-Party Data
Companies are increasingly focusing on zero-party data—information customers voluntarily provide. This approach improves data accuracy and supports stronger privacy compliance, while deepening trust and relevance in customer interactions.
- Edge Computing for Real-Time Processing
Edge computing accelerates data analysis by processing information closer to the source. This advancement enables immediate personalization in physical retail spaces and connected environments, removing latency from the equation.
- Predictive Segmentation in Voice and IoT
Voice-enabled devices and IoT ecosystems are unlocking new use cases for predictive segmentation. Businesses can now deliver context-aware marketing and services across these emerging channels, expanding the reach of personalization.
- Ethical AI and Explainable Models
As AI becomes central to decision-making, transparency is no longer optional. Companies are prioritizing ethical AI practices and developing models that can clearly explain their outputs to customers and regulators alike.
- Cross-Platform Unification
To meet rising expectations for seamless experiences, businesses are unifying predictive insights across all customer channels. This integration supports real-time, consistent engagement through a single, cohesive customer profile.
In conclusion, predictive segmentation continues to evolve into a critical capability for modern marketing. It allows businesses to shift from generic messaging to highly personalized engagement, grounded in real-time insights. Businesses that embrace advanced analytics, dynamic personalization, and ethical data practices can create experiences that feel relevant, timely, and customer-centric.
These efforts not only strengthen customer lifetime value and loyalty but also help brands stand out in a crowded marketplace. Those who lead in this space will gain a lasting competitive edge in the increasingly data-driven economy.
Shivkumar M 
Head Product Launches, Adoption, & Evangelism.Expert in cross channel marketing strategies & platforms.
Free Customer Engagement Guides
Join our newsletter for actionable tips and proven strategies to grow your business and engage your customers.
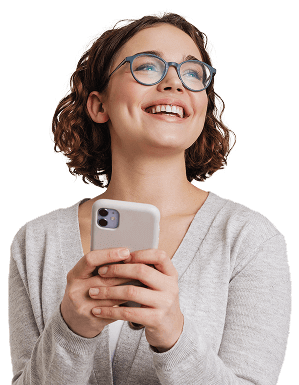