Is the purpose of analytics or data science to draw some insights from data or some cool visualization or is it just a recommendation based on some metric we deem important? The list could be endless. But, what is true analytics or data science?
Let’s begin with the definition of Analytics and Data Science given by Wikipedia.
Analytics: Analytics is the discovery, interpretation, and communication of meaningful patterns in data.
Data Science: Data science is an interdisciplinary field about processes and systems to extract knowledge or insights from data in various forms, either structured or unstructured, which is a continuation of some of the data analysis fields such as statistics, data mining, and predictive analytics, similar to Knowledge Discovery in Databases (KDD).
One common thing in the above definitions is to draw actionable/meaningful insights from patterns by means of data. Do we approach analytics or data science in a structured fashion to draw such actionable insights? Or Are we relegating analytics to an insignificant process within a larger project?
Analytics as a Project
Let’s not limit the scope of analytics or data science to a definition but rather, understand it from the point of view of a project with the help of a comparison:
The above comparison breaks an Analytics project into 5 stages broadly classified into:
i) Problem Statement
Quoting the Bible, it is said, “Ask and you shall receive”. So, be careful in what you ask for. Hence, asking the right question becomes important since the quality of the answer depends on it.
In analytics, not only asking the right question is important but also quantifying the question is equally important. This would result in defining the Problem Statement precisely.
Suppose you have a client meeting in 30 minutes, which involves travel from point ‘A’ to point ‘B’ in 30 minutes. You have the recourse to your colleague who is an expert in helping you to navigate the same. There are multiple ways to reach the above destination.
What would be the question to your colleague? Would it be:
a) What is the route to the destination?
b) How should I travel from point ‘A’ to point ‘B’?
c) How should I travel from point ‘A’ to point ‘B’ within the stipulated 30 minutes?
Clearly question (c) is the best question since you have not only mentioned the qualitative aspect of the question but also quantified it. Likewise, in any analytics project, it is important to clearly quantify your goals and not leave it open-ended.
For eg: Problem Statement 1: Increase Sales
Problem Statement 2: Increase Sales by 10%.
Clearly, Problem statement 2 is much clearer as it has quantified the problem statement and not left it open-ended.
ii) Data Collection
An analyst could get access to the required data at her disposal in varied formats: structured, semi-structured and/or unstructured. She may have to source additional data from external sources too. Depending on the scope & type of the project, the data might be suitably released or accessible to the analyst.
iii) Data Exploration and Data Preparation
The next logical step for an analyst is to start exploring & analyzing the data to get familiar with the inherent structure within the data. This may involve univariate and multivariate analysis of the variables along with visualization. One might encounter various anomalies in data, which might include missing data, erroneous data, outliers, etc, requiring the analyst to go back to the Data Collection Stage. The Data Exploration stage enables the analyst to form hypothesis to achieve the end goal of the analytics project.
Data Preparation follows Data Exploration where various anomalies are treated based on the level of importance and need. This may involve treatment or imputation of missing values, outliers, etc. One of the key ingredients in making the predictive models robust is Feature Engineering, which is simply a thoughtful creation of new input variables from existing input variables with valuable variables from domain expertise, logical reasoning, or intuition. It is during the Data Preparation stage where Feature Engineering is performed.
iv) Modeling
As an analyst, you would tend to have a hypothesis about the required independent variables & reinforced by the findings in the Data Exploration and Preparation stage to achieve the end goal of the project. Based on such a hypothesis, one would then test and create a predictive model. The predictive model could be built for regression, classification, clustering, segmentation, etc. This stage may involve numerous iterations using Feature Selection techniques and most often, an analyst tends to go back to the Data Collection, Exploration and Preparation stage, in order to make the model robust.
v) Presentation
Communicating and utilizing the insights and the predictions drawn from the Modeling stage is the next step. It is not just enough to make predictions but it is equally important to communicate the results, reasons and the steps required to act upon those predictions. Often the analyst may have to present his findings to a non-technical audience. Similar to a doctor, an analyst not only needs to diagnose the problem but also, prescribe a solution for the diagnosed problem, which is the scope of Prescriptive Analytics.
For example: Suppose you are a marketing analyst and your goal is to segment and identify likely transacting customers. You won’t be just stopping after building a predictive model that predicted the likely transacting customers. In fact, you would tend to go after those customers who were predicted to transact but didn’t transact in reality and design appropriate campaigns based on their likelihood or probability to transact. This way you could create a highly contextual and personalized campaign that could drive conversions.
The role of the analyst may not end just here. She may also be involved in implementation of the recommended steps and monitoring the future intended outcome.
Closing Thoughts
In this article, we have discussed the various stages in an Analytics Project to understand the deeper meaning of Analytics and Data Science. The mandate of an analyst could encompass either all or a subset of the stages discussed above depending on scope, need and goal of the project. The various stages of an analytics project are inter-connected and the project itself could be an ongoing project where the analyst goes back to the modeling stage or stages preceding it in light of various scenarios like change in data structure, change in variables, deteriorating model performance, etc.
Jacob Joseph 
Heads Data Science.Expert in AI, Data & Analytics and awarded 40 under 40 Data Scientists in India.
70,000+ Marketers & PMs Trust Us
Join our newsletter for actionable tips and proven strategies to grow your business and engage your customers.
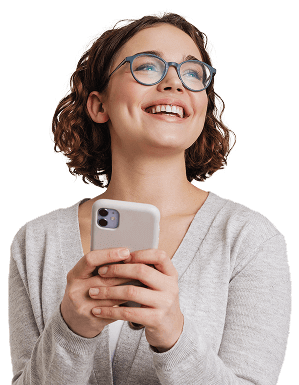