Never before have marketers had access to more customer data. User profiles can go beyond basic name and demographic data to include device preferences, social posts, browsing and content history, hobbies and interests, and much more.
In theory, marketers should have a near perfect understanding of their customers and target audience, as well as the best ways to engage them.
In practice, all that data means a lot of confusion. Creating user segments, identifying the right communication strategy for each, building relevant content and campaigns — marketers can’t succeed without mastering massive amounts of data.
But with machine learning and artificial intelligence, marketers can actually access and apply all that customer information to make meaningful connections with their audience.
Ready to welcome your marketing robot overlords? Keep reading to learn how machine learning and AI marketing helps today’s teams make smarter decisions, faster. We’ll also cover machine learning examples as well as predictive analytics examples to show how leading brands are integrating these cutting-edge technologies into their campaigns.
What Machine Learning Means for Marketers
The terms machine learning and artificial intelligence carry some heavy implications, from the utopian to the apocalyptic. Everyone from Bill Gates to Elon Musk has weighed in on the potential for these applications.
So what’s all the hype about? What is machine learning, exactly?
Simply put, machine learning is a subset of artificial intelligence. It gives computers the ability to learn from data and create accurate predictions — without explicit programming.
When it comes to the marketing world, the promise of machine learning is in quickly finding hidden patterns in massive amounts of consumer data. In fact, 97% of leaders believe that the future of marketing lies in digital marketers working with machine learning tools.1
Machine learning tools can effectively analyze what type of content, keywords, and phrases are most relevant to your target audience. They can create personalized content drips for each prospect and customer — and they can learn what’s most effective for each one in order to shorten sales cycles. In short, they can help marketers be more efficient and effective in nearly every aspect.
And it’s not just a possibility of the distant future. 84% of marketing organizations are already implementing or expanding AI and machine learning in 2018.2
Here are 6 ways you can apply the power of machine learning and artificial intelligence to your marketing campaigns right now.
1. Segmenting Customers with AI
When every user generates hundreds of data points, manual segmentation gets cumbersome fast. What qualities or actions do you segment on? How many factors do you include?
Predictive analytics can cut out guesswork and quickly identify key user segments. Think those most at risk of churn or those most likely to convert. Best of all, it automatically updates segments to provide a real-time look at your user base. This ensures that every user gets the right message at each stage of the customer journey.
But beyond that, ML-powered segmentation can analyze your user base to find complex patterns and correlations. Which lets you take personalization to the next level.
Take fitness brand Under Armour. They’re using machine learning in their Record fitness app to automatically identify precise user segments.3 They then match those segments to successful customers to create highly customized fitness recommendations.
The app pulls a user’s health data from third-party apps, smart watches, and data entered by users. It also factors in details like nutrition, sleep patterns, and workout stats to get a complete user profile. It then matches users to others with similar health and fitness profiles to offer coaching recommendations based on what’s proven to work.
Image: UA Record
The more users engage with the app, the better its machine learning algorithms get. It can match users to provide customized — and super effective — fitness recommendations.
2. Predicting Customer Behavior
What if you could anticipate what your users will do next?
By analyzing customer data, ML-based prediction algorithms can extrapolate future user paths. It can pinpoint users on the path to churn before they uninstall or identify users primed for conversion. With these insights, marketers can prevent churn or run targeted promotions that yield better ROI.
Amazon has been using prediction models for years to refine its famous product recommendation engine, which now drives 55% of sales.4 But that’s not all their prediction model is good for. It also helps the retail giant forecast product demand and optimize inventory. This is especially valuable for seasonal or trend-based supply decisions.
Luxury luggage brand Tumi has also embraced machine learning to predict purchases and optimize the in-store experience.5 Store employees can now access a customer data platform that shows detailed browsing and purchase history, email activity, and recent search behavior.
Charlie Cole, Tumi’s chief digital officer, explains the power of AI to Digiday: a store manager who had worked with a specific customer for years tried out a new product recommendation tool that shows items the customer is most likely to buy. The tool suggested all women’s products. The manager assumed this was wrong, since the customer had only purchased men’s accessories. So he called the customer to ask what he was currently shopping for, only to discover he was looking for a gift for his wife.
“We knew recent browser behavior, email open rates, search behavior — it’s far more predictive than past purchase,” said Cole. “What I bought yesterday isn’t always going to predict what I buy today. To get to that layer, you have to combine his purchase history with his browser behavior and email open rates. That’s what AI can do.”
3. Fine Tuning Cross- and Upsell Strategies
Perfecting your cross- and upsell strategy is like walking a tightrope. You need to increase purchase frequency and order values, but you have to do it in a way that’s not intrusive or annoying for shoppers.
Not to mention the massive amounts of purchase data you have to mine. You have to know which products are bought together, which products a certain customer profile typically buys, and which items shoppers are more likely to splurge on. And you have to pitch these products in a way that’s genuinely helpful to customers.
With machine learning, marketers can ensure data-based product recommendations reach the right customer on the right channel at exactly the right time. All in a way that actually enhances the customer experience.
Take Hyatt as an example. The hotel brand uses machine learning to analyze a guest’s travel history and accommodation preferences.6 Desk agents then automatically get an alert when the guest they’re checking in is likely to want to an upgrade or a room with a view. Or be interested in hotel amenities like spa or laundry services.
According to Hyatt’s SVP for Strategy and Analysis Chris Brogan, the program has increased the average incremental room revenue, post-reservation, by 60%.
4. Using RFM to Identify the Right Engagement Channel
RFM (Recency, Frequency, Monetary) Analysis is a powerful tool for identifying the best channel and time to engage key user segments.
Marketers can pinpoint users who will be receptive to an engagement campaign. And they can view the most effective channel for that segment. With this mix of data science and machine learning, marketers can deliver messages when users are most likely to convert, on the optimal device and channel.
Beauty brand Sephora uses this strategy7 to perfect its customer engagement campaigns. By analyzing members of their Beauty Insider customer loyalty program, they discovered that loyal clients make up 20% of their core customer base and spend the most money. They’re also the most active on social media, making them potentially valuable brand advocates.
Sephora also uses an AI-powered tool to pull detailed information about a customer’s profile and purchase history. This helps the company give shoppers a personalized experience that’s consistent online, on the app, or in-store. Product recommendations and tutorials can be tailored to skin type and shade, beauty routine, and past purchases. And Sephora can notify customers immediately via email, push notification, or SMS when their favorite products are back in stock or on sale.
5. Identifying and Acquiring High-Value Prospects
Using identification models, machine learning applications can find the prospects who are most likely to become customers. And not only that, by identifying prospects with attributes similar to existing customers, it can help identify those likely to become your most valuable customers.
By prioritizing the high-value prospects that are most likely to convert, brands can focus resources and optimize marketing spend.Mazda used this type of identification model to find the perfect social media influencers to promote the new CX-5 at the SXSW festival in Austin.8 Using IBM’s Watson AI technology to scan posts across major social media networks, the automotive brand identified artistic extroverts — the ideal persona to connect with the festival’s attendees.
Images: Mazda; Dan Hogman on Instagram*
Mazda selected four people to drive the CX-5 around Austin and hang out in the Mazda Studio, then share their experience with their followers. This data-centric approach to influencer marketing allowed Mazda to effectively connect with a highly creative audience.
6. Engaging Users with Intelligent Chatbots
When integrated with artificial intelligence, machine learning, and natural language processing, chatbots can be incredibly sophisticated tools for customer engagement.
AI-powered chatbots are programmed to learn from previous customer conversations so they can improve over time. They can understand complex requests, personalize their responses, and improve customer interactions over time.
And bots often provide the fastest possible time to resolution for simple customer queries. Of the 71% of people using messaging apps for customer assistance, many do it because they want an immediate answer.9
But bots aren’t just for solving simple support tickets. They can be a powerful user engagement tool.
Take popular language learning app Duolingo. They created a chatbot for users to practice their conversational language skills, judgement-free.10
The company originally paired users with native speakers for conversational sessions. But according to co-founder and CEO Luis von Ahn, 75% of them were too anxious to practice with another person. The chatbot offers a friendly practice partner, available 24/7, with no fear of embarrassment.
Image: Duolingo
It’s an effective tool to keep power users coming back to the platform to practice more advanced language skills in a convenient way.
Power Up Your Marketing Campaigns with Machine Learning
CleverTap uses industry-first machine learning features to process millions of data points across your entire user base, instantly connecting the dots between critical customer interactions.
Our advanced data science models help you group users by interest, identify the optimal channel and time to engage users, and deliver a personalized user experience.
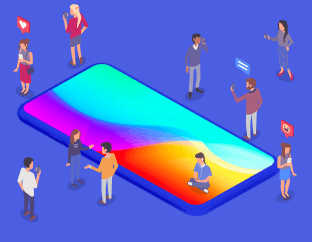
See how today’s top brands use CleverTap to drive long-term growth and retention
Shivkumar M 
Head Product Launches, Adoption, & Evangelism.Expert in cross channel marketing strategies & platforms.
Free Customer Engagement Guides
Join our newsletter for actionable tips and proven strategies to grow your business and engage your customers.
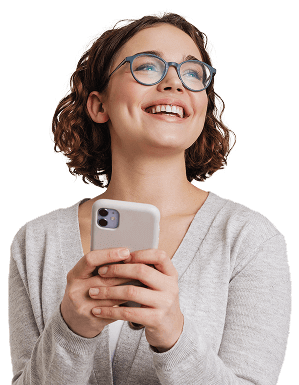